Neuromimetic sensorimotor processing
ESR5
Objectives
The tactile features that generate the space of tactile inputs correspond to specific contact conditions governed by contact mechanics encoded by the brain. At a fundamental level these features correspond to mathematical invariants which are hypothesized to be learned by the neural circuits of the brain, and for which we recently provided experimental evidence. In this project, we will use a (artificial) deep neural network to learn these invariants from neural recordings and data obtained from stimulating artificial skin.
Expected Results
Identification of neural coding invariants of haptic sensing.
Placement
Host institution: Actronika
Enrolments (in Doctoral degree): Lunds Universitet
Supervisors
Vincent Hayward, Etienne Burdet
Presentation of ESR5
PhD defence: May 7th 2024
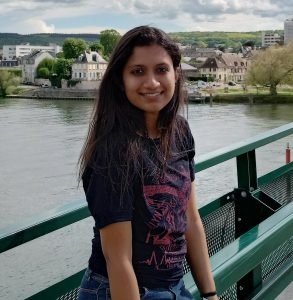
My name is Aruna Ramasamy. I am 23 years old. I pursued my undergraduate studies in Electronics and Communication Engineering in Anna University in my home country India. My inquisitiveness in Machine learning and Robots landed me in a master’s titled Computer vision, Robotics and Machine learning at University of Surrey in United Kingdom. I am always gravitated towards research as it provides a platform to explore and enrich one’s knowledge. Eventually, I found my way as a research student in the INTUITIVE project.
Abstract of PhD goals
Embarking on a scientific expedition to delve into the nuanced realm of tactile sensation, this thesis ventures into the domain of Neuromimetic Sensorimotor Processing- a field dedicated to bridging the divide between human sensory experience and artificial replication. At the core of this investigation is the endeavor to decode the language of touch, aiming to identify the so-called invariants that reveal the intricacies of texture felt by our fingertips. These invariants are stable features of tactile data that persist through various exploration conditions of touch, that can help us to reveal the brain’s method of recognizing and distinguishing between tactile sensations. While progress has been made in interpreting tactile data through vibrations, our understanding of key features that facilitate the tactile exploration of textures is unknown. This research seeks to identify representations that both simplify tactile data and preserve essential elements for decoding tactile perception. Through the lens of artificial intelligence and deep neural networks, this thesis delves into the untapped aspects of tactile perception, seeking to unveil the mathematical invariants that maintain mathematical consistency across variations in touch dynamics. To explore this theory, the research adopts a detailed methodology focused on gathering tactile data directly from the interaction with textured surfaces. This data collection encompasses friction-induced vibratory signals and other pertinent measurements, such as acoustic emissions, force feedback, and spatial data, all obtained during the process of tactile engagement. These invariant features are expected to be instrumental in the accurate categorization of a wide array of textures, illustrating the effectiveness of a custom-built machine learning architecture in emulating the brain’s aptitude for sensory input processing. This layered approach facilitates a comprehensive analysis, demonstrating the significance of audio-inspired features, particularly Mel-Frequency Cepstral Coefficients (MFCCs), in distinguishing between textured surfaces. The study’s focus on MFCCs, especially their extraction and performance within autoencoder models, underscores their value in effectively differentiating textured surfaces, even from a compressed representation of data. The conclusions of this research carry profound implications for the development of tactile sensing technologies and their application in creating more dynamic and immersive tactile experiences. The findings enable the development of innovative approaches in modality conversion, leveraging these mathematical invariants aiming to enrich auditory experiences with integrated haptic feedback in settings like music enjoyment or interactive VR experience.
Results
Deliverable 3.1 Learning classifier system for high-dimensional sensorimotor data
Characterization of neuromimetic sensorimotor processing. Identification of tactile interaction invariants. Implement learning classifier system for high-dimensional data
Conference Article
Ramasamy, A.; Faux, D.; Hayward, V.; Auvray, M.; Job, X.; Kirsch, L.
Human Self-touch vs Other-Touch Resolved by Machine Learning
EuroHaptics, 2022
DOI: 10.1007/978-3-031-06249-0_25